The evolution of AI-related services is starting to reshape demand for high-speed, high-capacity, low-latency fibre networks. Here’s the state of play as we see it.
For many people, AI is still a novelty. You only have to look at the recent meme craze sparked by OpenAI’s Studio Ghibli-style image generator to appreciate that in mass-market terms, generative AI is still in its infancy.
But behind the scenes, AI is evolving and maturing—both in its use cases and in the services that support them. Many use cases involve large quantities of data or real-time responsiveness, and that’s starting to have a profound impact on demand for network speed, bandwidth and latency.
In this blog, we look at four emerging AI-related services, and how resilient connectivity underpins the success in a world ruled by distributed cloud-based systems.
Four new AI services—and their connectivity implications
The evolution of data-intensive AI use cases is spurring the introduction of new services by incumbents and startups alike. In our work with companies in the AI space, we’re seeing four new service types emerging.
1. Real-time model training
Today, large language models (LLMs) like GPT-4, Llama and Claude have been pre-trained before being deployed to developers and users—in fact GPT stands for generative pre-trained transformer. Gathering training data for these models isn’t time-sensitive, and the training is completed offline.
Now, some services are moving to a real-time approach, where data is continually collected and used to train the model on the fly. Real-time training may have applications in sectors where informational inputs to decision-making are changing quickly—such as defence, public health or banking.
The impact on bandwidth requirements here is potentially colossal, depending on the volume and nature of the data being gathered, and the location of the data and of the model being trained. A strategic approach to connectivity and trusted, flexible connectivity partners will be essential.
2. Inference clusters
Inference clusters represent deployments of a particular pre-trained LLM for a specific use. An example might be a retailer’s customer service chatbot that uses GPT4 as its model. Inference is the work done by the pre-trained LLM to generate relevant responses to a customer’s query.
Growing use of LLMs makes it expensive from a data transport point of view to conduct all of the inference work in the same physical location as the model—for example, if the model is hosted in the US but the users are in Europe.
There are also expectations that the inference engine will respond in real time to users. And depending on the industry, regulators also may demand that user data stays in Europe, precluding the use of a US-hosted model.
One solution is to build inference clusters: localised deployments of the model that keep user data local, require data to be transported over shorter distances, and respond faster due to lower latency.
Companies deploying geographically-distributed inference clusters need assured high-bandwidth, low-latency connectivity to, from and between each cluster, to keep models in sync and up to date, to handle a growing user base, and to cope with unpredictable peaks in usage.
3. Neoclouds
Growing demand for inference clusters and other distributed LLM deployments is driving the emergence of a new type of infrastructure provider. Since LLMs require powerful GPUs to run, we are seeing the rise of ‘neocloud’ companies dedicated to offering GPU resources as a service (GPUaaS).
Operating on a similar consumption-based model to the hyperscalers, neoclouds are geared to handling AI workloads specifically—from model training to inference and predictive analytics. Digital Ocean, for example, is offering bare-metal GPUs to AI-intensive European companies from its data centre in Amsterdam.
The Uptime Institute has found that in many cases, neoclouds can be more cost-effective to use than GPU instances offered by hyperscalers, a sign perhaps that rapid growth is on the horizon. Indeed McKinsey says that 70% of data centre demand is for ‘infrastructure equipped to host advanced-AI workloads’.
But offering GPU resources is only part of the story. Neocloud providers will also need to meet user expectations for speed and latency while keeping services at an attractive price point. That will mean thinking strategically about connectivity—identifying suitable locations and potentially developing partnerships with AI-savvy connectivity providers, rather than buying capacity off the shelf.
4. Enhanced and micro data centres
Neoclouds aren’t the only infrastructure providers stepping up to the AI age. Traditional data centres and hosting providers are reconfiguring their operations to cater to AI workloads too.
Zayo Europe customer Lunar Digital is one data centre and colocation services company that’s investing in new capabilities to support AI-intensive use cases—from healthcare diagnostics to financial fraud detection. It’s currently re-engineering the cooling infrastructure at four Manchester data centres to support high-density GPU deployments.
“It will enable businesses to unlock AI’s transformative potential without the power and cooling constraints of conventional colocation environments,” CEO Robin Garbutt told us.
Companies that operate a distributed footprint of micro data centres, meanwhile, may be well placed to meet the latency demands of real-time AI workloads. With the right infrastructure in place to connect edge data centres to each other and to core data centres and transport networks, micro data centre operators may gain an edge over more centralised competitors
As an example, Zayo Europe customer Pulsant has 12 edge data centres strategically located around the UK, including Edinburgh, Manchester, Rotherham and Newcastle. All 12 are interconnected by Zayo Europe’s low latency network with access to our Tier 1 global Internet backbone—meaning Pulsant can serve low-latency use cases by avoiding congested backhaul routes through London.
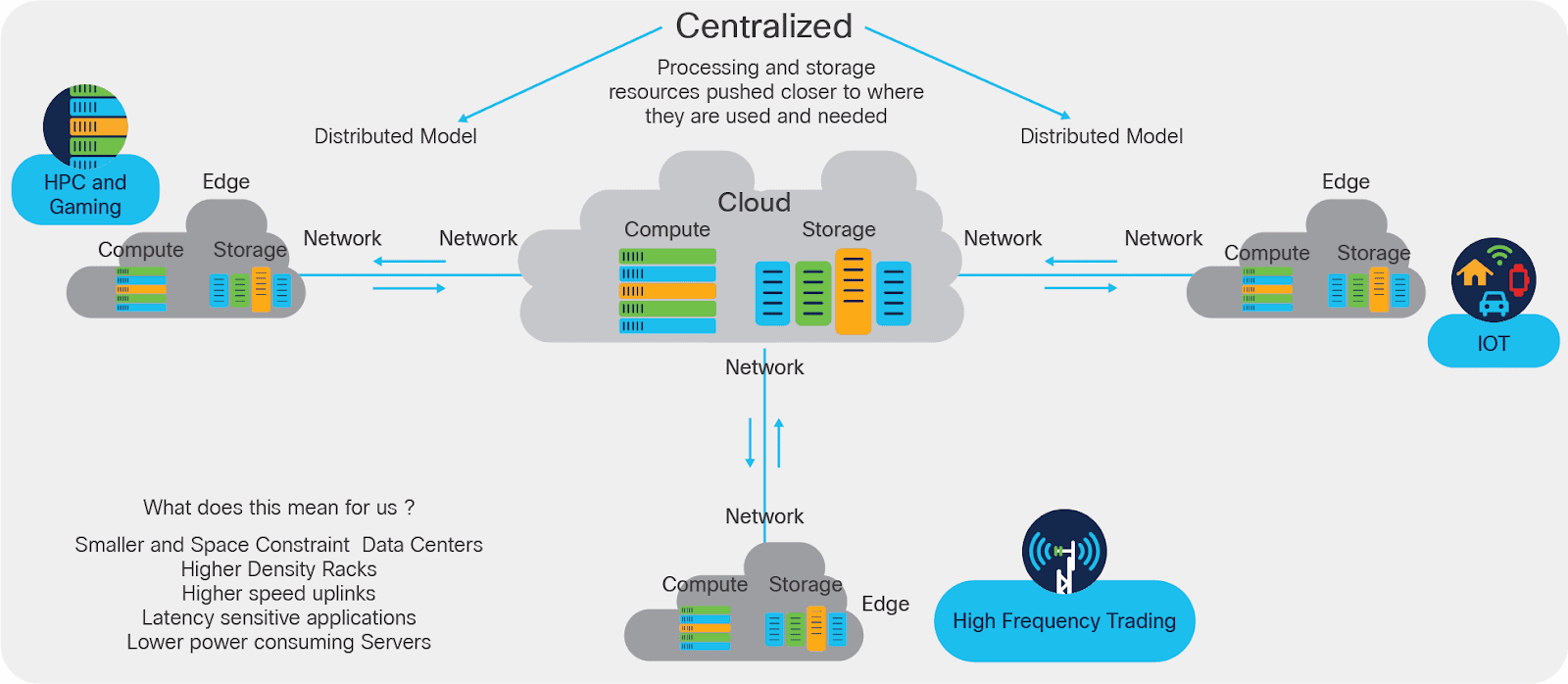
Talk to Zayo Europe about connectivity for AI services
The evolution of AI is already having a profound impact on demand for ‘resilient’ connectivity. This will only intensify as more capacity, faster speeds and lower latency are needed to meet customer demand.
Zayo Europe is already working with companies across Europe to ensure they have the right connectivity for their current and future AI use cases. From 400G wavelengths to private wavelength networks and dark fibre for both long-haul and metro connectivity, we have a solution that can support your AI business today and into the future. If you’d like to know more, please get in touch.